Blog Post
OCR (Optical Character Recognition) is a technology that automatically converts handwritten, typed, or printed text into a digitally-encoded, editable, machine-readable format. Despite its market share being projected to hit an all-time high over the course of the decade, questions remain about the true practicality and business value of OCR software.
Through a combination of AI, automated data capturing and storage, and occasionally specialized hardware, optical character recognition can yield massive efficiency, accuracy, and revenue gains by automating document processing.
One organization may be faced with mountains of purchase orders, invoices, and customer data documentation that require a human employee to extract important data manually; on the other hand, another organization, one which has implemented OCR, could have those same documents digitally recast in a machine-encoded text file – with greater processing speed, improved accuracy, and enhanced data flexibility, as well.
This potential to exponentially boost efficiency across high-value but low-skill workflows is one of the great appeals of OCR.
Growing OCR adoption in the manufacturing and distribution sectors has triggered unprecedented market growth, valued at over $10 billion in 2022 and forecast to expand with a CAGR of 15.4% until 2030.
However, there is a caveat that is important to factor in from the outset. OCR is not without its limitations.
Many businesses experience ongoing frustrations applying OCR to a ‘real-life’ scenario. One common example is OCR’s inability to process certain languages, fonts, or handwriting styles accurately. This accuracy gap represents a significant operational risk for businesses.
In this article, we’ll inspect the systems by which OCR operates and discuss its functionality. We’ll then consider OCR use cases, benefits, and drawbacks, as well as what the future of OCR might look like as emerging alternatives with better accuracy grow in popularity.
How OCR Works
In the most simplistic terms, OCR technology works according to the following steps:
1. Source document
An organization identifies the source document – trade contracts, purchase orders, invoices, service agreements, handwritten documents, legal paperwork, and even a photograph or historical document – containing the desired information.
2. Scanning
The source document (or image) is scanned to create a digital file such as a PDF or a JPEG. While some OCR software can scan documents independently, others will need the operator to manually scan the source document and feed it the resultant file.
3. Identification & recognition of characters
This is when the OCR system executes the most important part of its function. Using one of several possible processing models, the software identifies characters within the document – recognizing the words, figures, spaces, line breaks, and ultimately the entire body text, along with headers and captions, that make up the scanned document.
4. Machine-readable text file
Having recognized the scanned text, OCR systems transmute the data into a digitally-encoded text file. This file is storable, searchable, and editable for business staff within a centralized system of records and should, at least in theory, be accurate to the original.
Step 3 of the above process, the identification, and recognition of contained characters, relies on the technology being capable of effectively and accurately analyzing areas of light and dark on the document. It will then factor in various rules to assist that processing – allowances made for variant typefaces or translation, for instance – to arrive at the machine-encoded text file.
Early OCR systems only worked with documents formatted in a single, specific font designed for that express purpose. Nowadays, OCR systems are much more flexible, some can recognize individual handwriting styles in multiple languages. This refined technology is known as ICR (intelligent character processing).
While some systems do not enable the selectivity to pick and choose which areas of the document are to be scanned, others are capable of zonal OCR. This allows users to set parameters and margins within the document, and the software will not include any data beyond those boundaries in the processing.
Zonal OCR can decrease the chances of character misidentification and slightly accelerate the process overall.
With OCR, there are two solutions for processing characters:
Pattern Recognition
Were every document composed in a single, standardized font, the concept, and execution of OCR would be vastly simplified; there would be no discrepancy between the same characters in multiple documents.
For this reason, in the 1960s, early OCR engineers developed a specialized font known as OCR-A. With every character single-spaced or regularly-sized, and the stroke composition of each character designed to be identifiably distinct from others, OCR technology had a much easier time processing documents.
However, this proved to be an unworkable solution in real terms. There was, and is, no practical method of procuring every document in standard OCR-A. Moreover, pattern recognition OCR would never be able to deal with handwritten text.
The next step in pattern recognition development was to ‘teach’ OCR to recognize the most commonly-used fonts, such as Arial, Times New Roman, and Helvetica. This, though, increased the risk of errors and inaccurate processing.
Feature Detection
Sometimes referred to as feature extraction or ICR (intelligent character recognition), feature detection is a more refined and sophisticated method of processing characters.
It uses character composition rules to adaptively identify text, regardless of font or handwriting style. For instance, an OCR platform would be programmed to recognize two diagonally intersecting lines that meet in the middle as an ‘X,’ with a larger character indicating uppercase and smaller characters indicating lowercase.
In this way, the OCR platform can theoretically recognize any source document with reliable accuracy.
Most omni-font OCR systems capable of recognizing information in any typeface employ feature detection, whilst some use neural networks, which are software platforms designed to identify patterns in a way similar to the human brain.
Why Implement OCR? Use Cases & Benefits
For organizations, OCR is mostly employed to capture and convert information contained within paper (or inconsistently formatted) documents. In this way, the organization can digitize its records and boost the efficiency of processing data.
Businesses may look to implement big data, where digitally-encoded documents can be referenced and worked alongside data sets from multiple other sources. This, theoretically, eliminates the need for any manual data handling.
OCR has also found several practical applications throughout society in general. It has been deployed as an aid for the visually impaired or in text-to-speech software.
Many translation services leverage the capabilities of OCR; furthermore, numerous scenarios exist where the large-volume scanning of standardized characters is necessary – passport control or license plate recognition, for example – and OCR may be applied here to great effect.
For an enterprise, the core benefits of optical character recognition include:
- Enhanced data accuracy
- A boost in productivity
- Lower cost of operations
- An elevated CX (customer experience)
- Increased data security
For more detail on the business advantages of OCR, read our blog post, ‘Defining 7 Business Advantages of Optical Character Recognition.’
The Limitations of OCR
Despite the above, OCR cannot be called a truly accurate, infallible data-extraction software tool. Because of the massive variation in character composition and languages, as well as the not-quite-complete data journey that OCR facilitates, it remains an inherently flawed system.
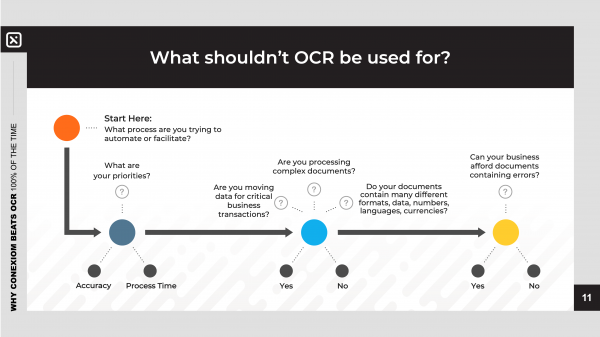
Source: https://conexiom.com/on-demand-content/why-conexiom-beats-ocr-100-of-the-time/
OCR does not complete the unstructured-to-structured data journey
OCR software, even if it does its character identification job perfectly, can only take an organization so far. While it will scan, analyze, and digitally encode information, companies will need to use bolt-on technologies – or a member of the human workforce – to go on and perform further work with that data.
Problematic formatting
Lookalike characters, such as ‘l’ and ‘1’ or ‘8’ and ‘B,’ are a major pain point for OCR software, and misidentification will lead to inaccurate or even nonsensical processing. This issue is compounded by the sheer variety of typefaces available and the significant disparity in handwriting styles. Moreover, pictographic or abjad languages have been noted as a marked difficulty for OCR processing.
Inevitable data inaccuracy
Whenever a business hands over control of its document processing to an OCR-powered platform, the risk of inaccuracies is a concern. OCR can never, in real terms, be 100% accurate 100% of the time. Certain elements within a source document, such as low quality, skewed orientation, or colored text/backgrounds will, eventually, be misidentified by OCR software.
OCR as a Component of Advanced Automation
OCR is receiving increased attention from business leaders because of its potential to integrate with AI. With the technology where it is now, the hope is that AI can step in to check and resolve any mistakes made by OCR to enable truly automated data processing.
Information Age describes AI/OCR tools as “sleeping giants in the wider topic of digital transformation.” There is, undoubtedly, a great demand for technology that can quickly process operation-critical information and simultaneously check, resolve, predict and navigate issues as and when they appear.
Conexiom: More Accurate Document Processing
Owing to the limitations and flaws of OCR that we’ve already seen, it remains an unsuitable document processing software for organizations aiming at truly accurate document processing. Digital transformation and genuine hyperautomation, at least at present, cannot be reliably supported with OCR alone.
Rather, we deploy patent-pending intellectual property to ensure that data is captured and transformed with 100% accuracy. Our system maps the content of a customer’s PO, paying homage to customizable business logic dictating exactly how that mapping should occur.
Request a demo today to discover the potential efficiency gains your business could yield through a partnership with Conexiom.